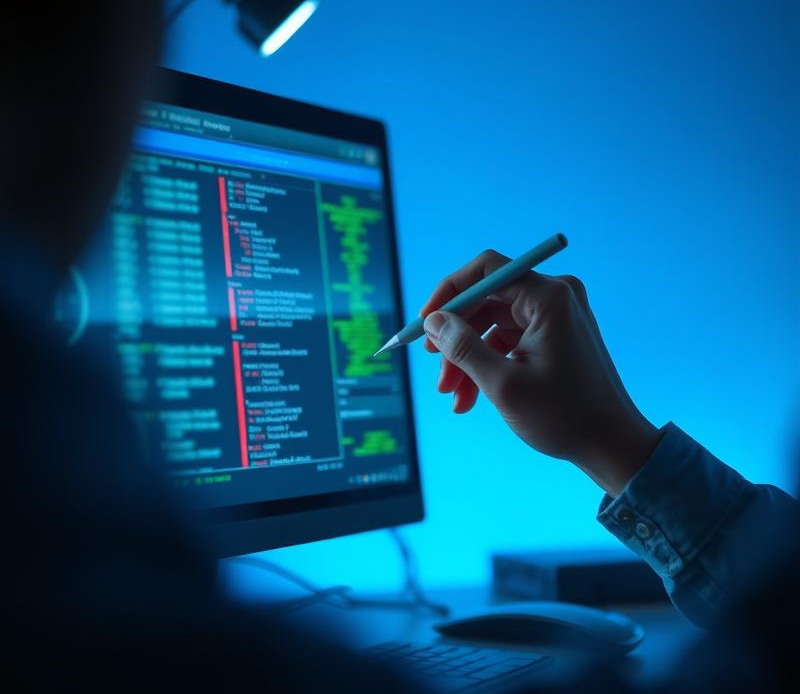
Why Accurate Annotation Matters in AI Development:
Data annotation for AI models is the process of labeling data to make it understandable for machines. This process is a fundamental step in supervised machine learning, where algorithms learn to make predictions or decisions based on labeled examples. Just like a child learns to identify objects by being shown labeled pictures, AI models rely on annotated data for machine learning to understand and interpret the world.
The Impact of Poor Data Quality on AI Models:
The accuracy and reliability of AI models are directly dependent on the quality of the data they are trained on. Inaccurate or inconsistent annotated data for AI models can lead to poor model performance, biased outcomes, and unreliable predictions. Imagine an image recognition model trained on poorly labeled images – it might misclassify objects, leading to errors in applications like self-driving cars or medical diagnosis.
The Role of Data Annotation in Machine Learning:
Data annotation in machine learning is not merely about adding labels; it’s about adding the right labels with context and precision. Different types of data require different annotation techniques. For instance, image annotation for AI might involve drawing bounding boxes around objects, while NLP tasks might require tagging parts of speech or identifying sentiments in text.
High-quality training data is the foundation upon which successful AI models are built. By investing in accurate and relevant data annotation for AI, businesses can ensure that their AI initiatives deliver on their promises.
Want to learn more about the challenges of data annotation?