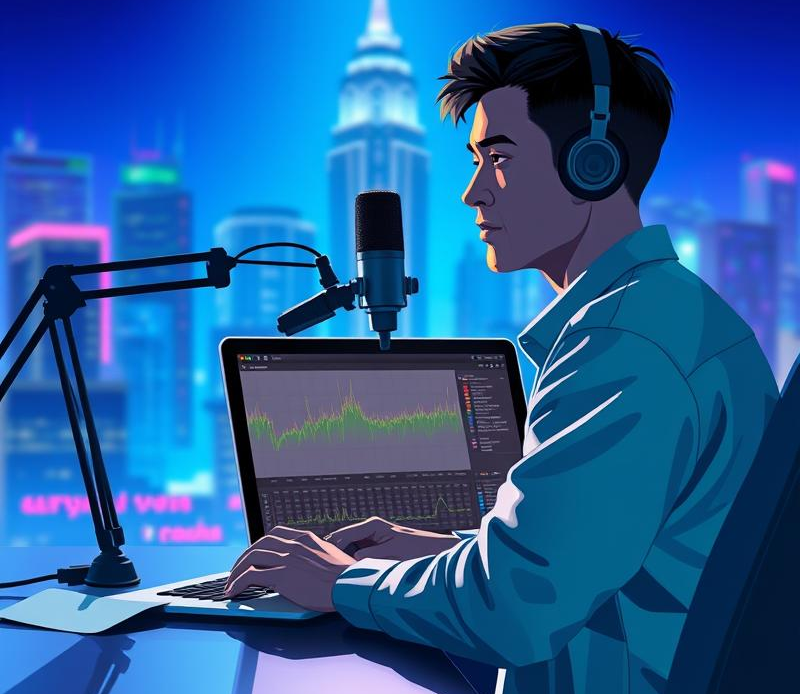
Master the art of perfect transcription with AI: improve accuracy, handle diverse audio, and unlock powerful applications.
From short clips to streaming input, explore customized solutions for all your audio transcription needs.
Introduction:
Ever felt like your voice just wasn’t being heard? You’re not alone. We all struggle with technology sometimes, especially when it comes to capturing the nuances of our speech. But what if there was a way to make your voice crystal clear, even in the noisiest of environments? That’s the power of advanced speech-to-text technology, and it’s about to revolutionize how we interact with the world around us.
- Training Custom Speech Models With Audio Data:
The world of AI audio transcription is constantly evolving, and one of the most exciting developments is the ability to train custom speech models. By feeding audio data into these models, either with or without human-labeled transcripts, we can achieve remarkable improvements in recognition accuracy. This is particularly useful for addressing specific speaking styles, accents, or background noises that might pose challenges for general-purpose transcription services.
For instance, imagine you’re developing a transcription service for a call center that handles a high volume of calls from a particular region. By training a custom speech model on audio data representative of that region’s accents and dialects, you can significantly enhance the accuracy of your transcriptions. This not only saves time and effort but also improves customer satisfaction by providing more reliable and understandable transcripts.
- Leveraging Domain-Specific Vocabulary Recognition:
Another compelling use case for custom speech data lies in domain-specific vocabulary recognition. Many industries and professions employ specialized jargon and technical terms that general-purpose transcription services may struggle to transcribe accurately. This is where custom speech models can truly shine.
By training a model on a corpus of text and audio data specific to a particular domain, such as medical, legal, or financial, you can equip it with the knowledge and context needed to accurately recognize and transcribe even the most complex terminology. This capability opens up a world of possibilities for businesses and organizations operating in specialized fields, enabling them to automate transcription tasks with a high degree of confidence in the accuracy of the results.
- Transcribing Short Audio Files: A Quick and Easy Solution:
For those who frequently need to transcribe short audio files, such as voice notes, short meetings, or interview snippets, synchronous speech recognition offers a convenient and efficient solution. This approach allows for the transcription of audio files up to a certain duration, typically around 60 seconds, in real-time or near real-time. Many transcription services, including cloud-based platforms like Google Cloud’s Speech-to-Text API, offer this functionality, often with a generous free tier to get you started.
This means you can transcribe a significant number of short audio files without incurring any costs, making it an attractive option for individuals, small businesses, and anyone looking for a quick and easy way to transcribe audio on the go. You can explore the capabilities of Google Cloud’s Speech-to-Text API and its free trial options on their website. For those interested in learning more about custom speech models and how they can improve speech-to-text accuracy, Microsoft Azure provides insightful information on their blog about enhancing speech-to-text accuracy with Azure Custom Speech.
- Tackling the Challenges of Long Audio File Transcription:
While synchronous speech recognition excels at transcribing short audio snippets, longer audio files, such as lectures, podcasts, or conference calls, require a different approach. This is where asynchronous speech recognition comes into play, offering a robust solution for transcribing audio files exceeding the typical 60-second limit of synchronous methods.
Asynchronous transcription processes audio in batches, allowing it to handle files of much longer durations, potentially hours in length. This method is particularly well-suited for scenarios where real-time transcription is not essential, and the focus is on generating accurate transcripts of lengthy audio recordings.
- Exploring Advanced Audio Transcription Use Cases:
The applications of AI audio transcription extend far beyond simple transcription tasks. One area where this technology is making significant strides is in the realm of machine learning model training. High-quality transcripts are crucial for training machine learning models in various domains, including natural language processing (NLP), speech recognition, and speaker identification.
By leveraging AI-powered audio transcription, researchers and developers can obtain accurate and consistent transcripts of large datasets, accelerating the training process and improving the performance of their models. This has far-reaching implications for fields such as conversational AI, virtual assistants, and other NLP-driven applications.
Moreover, accurate transcription plays a vital role in enhancing customer experience. For businesses that rely on phone calls, video conferences, or other forms of audio communication, transcripts provide a valuable record of customer interactions. By analyzing these transcripts, businesses can gain insights into customer sentiment, identify areas for improvement in their products or services, and personalize their interactions to better meet customer needs. Amazon Connect Contact Lens is one such service that offers powerful analytics capabilities based on transcribed audio data, demonstrating the transformative potential of AI audio transcription in elevating customer experience. For global businesses, accurate transcription combined with advanced translation services can break down language barriers and facilitate seamless communication with customers worldwide. Google Translate, with its extensive language support and sophisticated algorithms, exemplifies the power of AI in bridging communication gaps.
Conclusion:
So, there you have it! The world of audio transcription is evolving, and AI is leading the charge. Whether you’re a researcher, a business owner, or just someone who wants to make their audio files more accessible, there’s a solution out there for you. Don’t be afraid to experiment and see what works best for your needs. You might be surprised at how much easier it is to understand and utilize your audio data with the help of AI.