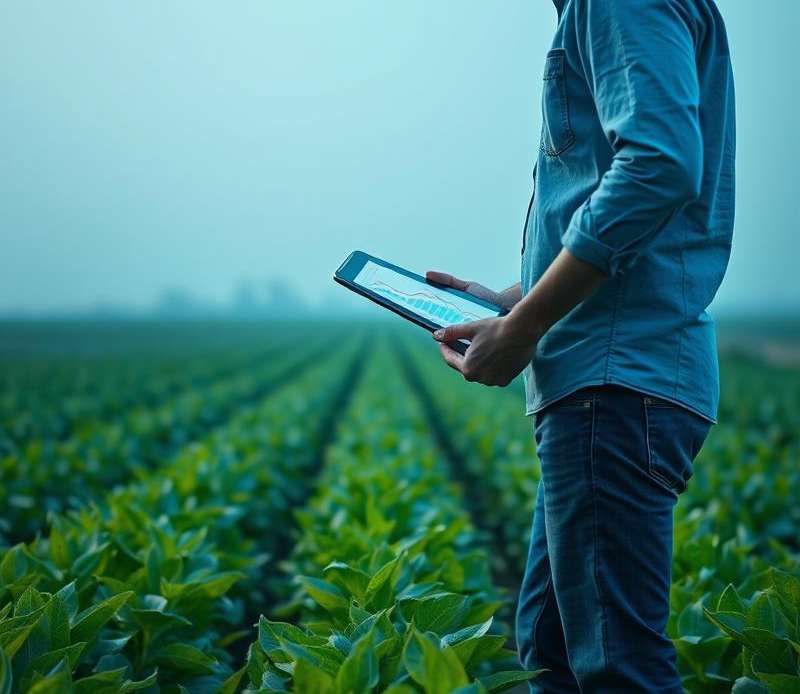
The agriculture industry faces a significant challenge in leveraging the vast amounts of data generated on farms for actionable insights. This inability to effectively utilize data hinders farmers from optimizing yields, managing resources efficiently, and adapting to changing environmental conditions. Addressing this challenge is crucial for global food security and sustainable agricultural practices. A practical solution lies in data annotation, a process that adds informative tags to datasets, enabling agriculture technology trends like artificial intelligence (AI) and machine learning (ML) to understand and analyze agricultural data.
Crop disease detection:
- Data annotation plays a vital role in training AI models to accurately identify and classify crop diseases from images.
- By annotating images of healthy and diseased crops, farmers can develop AI-powered systems for early disease detection, enabling timely intervention and minimizing crop losses. Learn more about how image annotation helps in developing AI crop harvesting systems.
Livestock health monitoring:
- Precision agriculture annotation extends to livestock farming, where data annotation helps monitor animal health and well-being.
- By annotating images and videos of livestock, AI algorithms can be trained to detect signs of illness, stress, or injury, allowing farmers to address potential health issues proactively. To learn more about annotating images in agriculture, read this complete guide.
Weed control and management:
- Data annotation is essential for developing AI-powered weed control systems.
- By annotating images of weeds and crops, farmers can train AI models to distinguish between them, enabling targeted herbicide application and reducing the reliance on broad-spectrum herbicides.