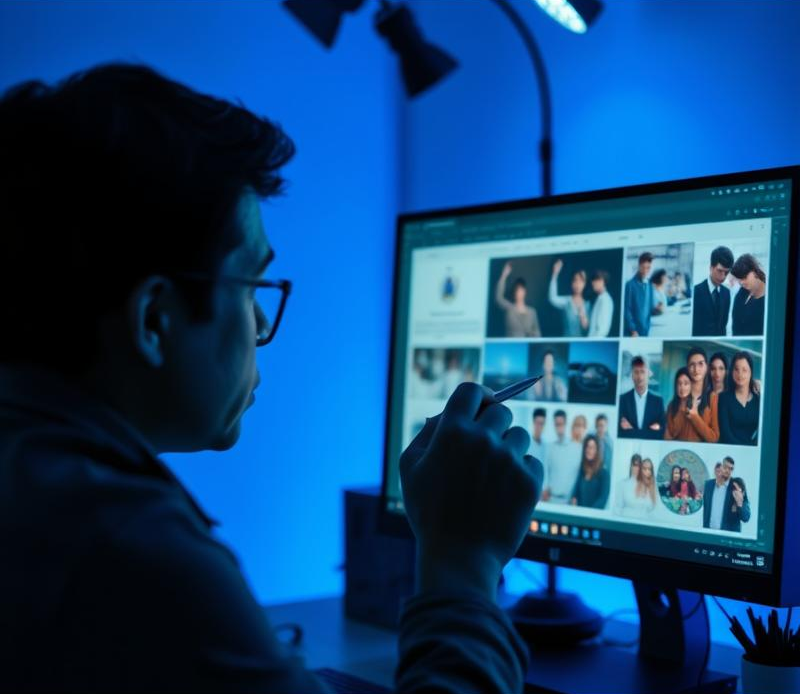
Common issues with image data annotation:
A frequent challenge in image data annotation is maintaining consistency. Inconsistencies arise from factors like human error, unclear guidelines, or variations in image quality. Another difficulty is the time and effort required for manual annotation, especially for large datasets. This process can be tedious and prone to errors, impacting the project’s timeline and budget.
Impact of poor annotation on machine learning models:
The accuracy of your machine learning models hinges on the quality of your image data labeling. Inaccurate or inconsistent annotations can lead to poorly trained models that make inaccurate predictions. This is because your model learns from the data you feed it; if the data is flawed, the model’s performance will be too.
Why image annotation is crucial for accurate results:
Image annotation is the foundation of computer vision applications. It provides context to images, enabling machines to “understand” the content. This understanding is what allows machine learning models to perform tasks like object detection, image classification, and more. Accurate annotations are vital for training robust and reliable models.
Curious to learn how image annotation fuels the engine of machine learning?