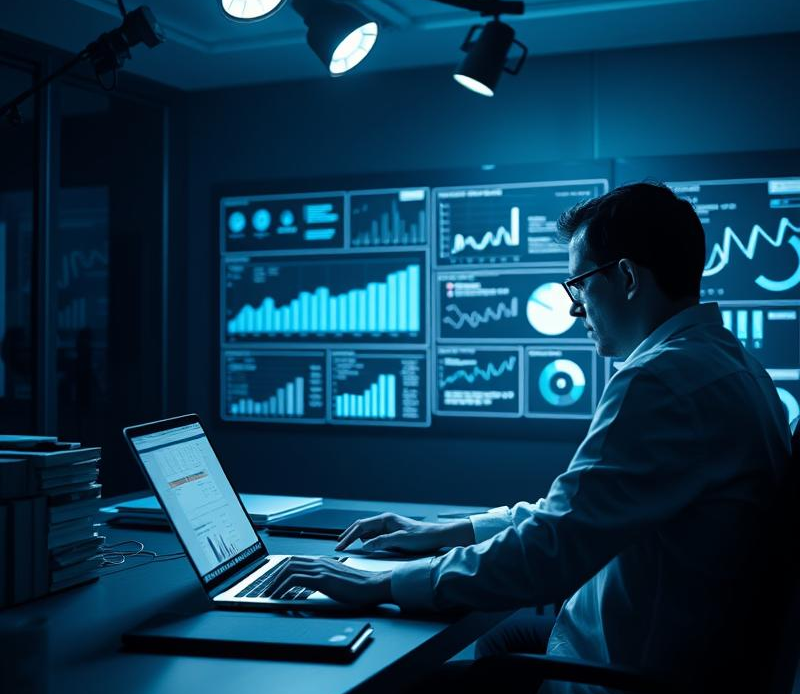
Transform audio into valuable data insights for AI and machine learning. Explore the power of accurate speech-to-text conversion for enhanced model training and analysis.
Introduction
Ever felt frustrated trying to make sense of audio data? It’s a common challenge in the world of AI and machine learning. Getting accurate transcriptions can be a real headache, especially when dealing with complex language or noisy environments. But what if you could unlock the power of audio data with high-quality transcriptions? This blog explores the crucial role of audio transcription in AI/ML, the challenges it presents, and how to overcome them for better results.
Understanding Audio Transcription for AI/ML
Imagine teaching a computer to understand human language. It’s no easy task. Audio transcription plays a critical role in this process, converting spoken words into text that AI and machine learning algorithms can process. This text data becomes the foundation for training robust AI models capable of understanding and responding to human speech. The more accurate the transcription, the better the AI model’s performance.
The Importance of Accurate Audio Transcription
Accurate audio transcription for AI/ML data analysis is paramount. Why? Because AI models learn from the data they are fed. Inaccurate transcriptions introduce errors into the data set, leading to skewed analysis and unreliable outcomes. For instance, if an AI model trained on medical transcriptions encounters numerous errors, it might misdiagnose patients or provide incorrect treatment recommendations.
Challenges in Achieving High Accuracy in Speech-to-Text Systems
While speech-to-text technology has advanced considerably, achieving high accuracy remains a challenge. Several factors contribute to this difficulty, including:
- Speaker Variability: Accents, dialects, and speech impediments can significantly impact transcription accuracy.
- Background Noise: Ambient sounds interfere with speech recognition, making it difficult for systems to distinguish between speech and noise.
- Contextual Understanding: Human language is nuanced. A single word can have multiple meanings depending on context, and speech-to-text systems often struggle with such ambiguities.