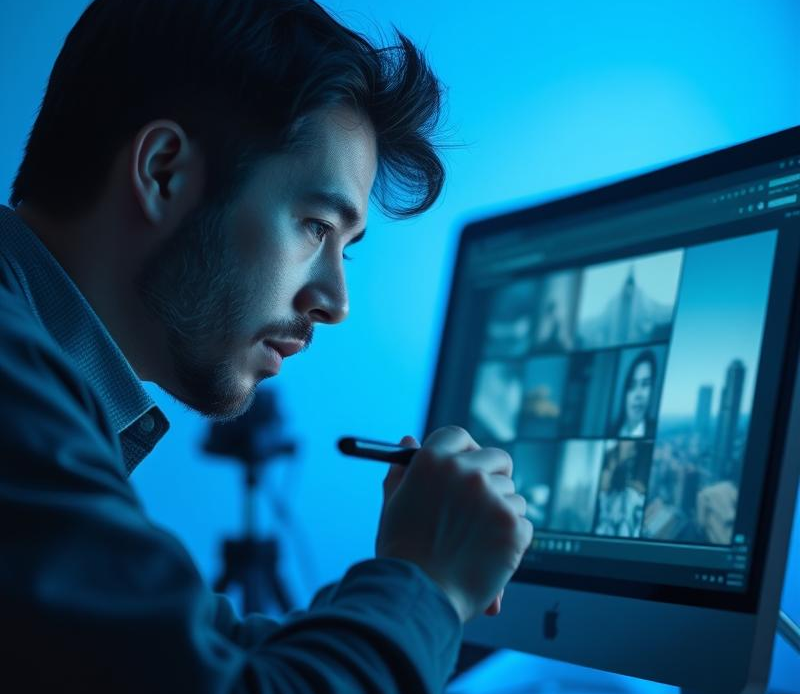
Employing Expert Annotators:
In the realm of computer vision, the quality of your image data annotation can directly impact the performance of your AI models. Inaccurate or inconsistent annotations can lead to flawed insights and unreliable predictions. One effective approach to ensure high-quality image annotation for computer vision is to employ expert annotators. These individuals possess domain expertise and a keen eye for detail, enabling them to provide accurate and consistent labels for your image data.
Establishing Clear Annotation Guidelines:
Clear and comprehensive annotation guidelines are paramount for maintaining consistency and accuracy in image data labeling. These guidelines should outline specific labeling conventions, object definitions, and edge cases to minimize ambiguity and ensure all annotators are on the same page. Well-defined guidelines act as a roadmap, guiding annotators towards producing high-quality labeled datasets.
Maintaining Iterative Review Process:
A robust quality control process is essential for identifying and rectifying any errors or inconsistencies in your annotated data. Implementing an iterative review process, where senior annotators or quality assurance specialists verify the work of others.